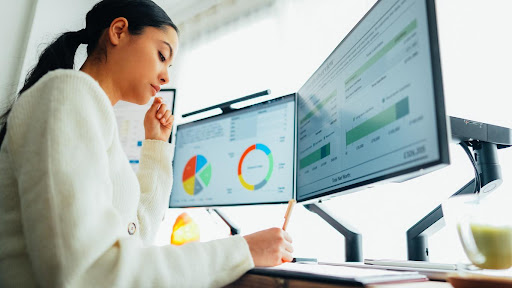
To remain relevant in today’s fast-paced, data-driven business landscape, being able to extract meaningful information from vast amounts of data is no longer optional. It is mandatory.
Data analytics have long been the cornerstone of successful decision-making. If you’ve been keeping tabs on trends, you must have noted that technological advancements like artificial intelligence (AI), automation, machine learning, etc. have elevated the potential of data analytics.
Today’s guide will explore how these advancements will reshape data analytics in 2023. Importantly, it will also touch on their impacts on businesses. Read on!
How has technology changed data analytics?
To understand the transformative power of today’s data analytics landscape, let’s first take a look back at its evolution. Traditional analytics methods relied heavily on manual processes and limited computational capabilities.
Nevertheless, they laid the foundation for data analysis in businesses. There has been a seismic shift in how organizations harness the power of data with the advent of AI, automation, and machine learning. We’ve rounded up below some of the key technological advancements and how they’ve changed data analytics:
Artificial intelligence (AI)
Artificial intelligence (AI) enables computers to mimic human intelligence. Thanks to it, it has emerged as a game-changer in data analytics.
Also, because of this capability, AI now empowers businesses to analyze vast volumes of data with unprecedented speed and accuracy. AI algorithms cannot just recognize patterns.
They can also make predictions and learn from data, leading to valuable insights and more informed decision-making. Today, we see AI-powered analytics tools and platforms revolutionizing various industries.
For example, in healthcare, AI algorithms can analyze patient data to identify rare diseases as well as undiagnosed and less-diagnosed patients. In marketing, AI-driven customer segmentation helps businesses target specific audiences with personalized campaigns. The possibilities are vast, and AI continues to unlock new levels of data analytics potential.
Automation and machine learning
Automation and machine learning go hand in hand with AI. They leverage algorithms to automate repetitive tasks and learn from data without explicit programming.
In data analytics, automation streamlines the entire process. From data collection to analysis, it handles almost everything, thus reducing manual effort and minimizing errors.
Machine learning, a subset of AI, enables systems to automatically improve and learn from experience. It uses sophisticated algorithms to uncover hidden patterns, detect anomalies, and generate valuable predictions.
Businesses can use these insights to optimize operations and identify trends. And what’s more, they can actually rely on these insights to predict future outcomes. For businesses that have embraced automation and machine learning, this gives them a significant competitive edge.
Predictive analytics
Predictive analytics uses historical data and machine learning algorithms to forecast future trends and outcomes. This is how it takes data analysis to the next level.
Businesses can anticipate customer behavior. And it doesn’t even end there. They can also anticipate market trends and potential risks by analyzing patterns and relationships within the data.
For instance, predictive analytics models can help institutions identify potential fraud or assess credit risk in the financial sector. In manufacturing, predictive maintenance can optimize equipment performance by proactively predicting failures and scheduling repairs.
Natural language processing (NLP)
Natural language processing (NLP) enables computers to understand and interpret human language. In data analytics, it’s key to extracting insights from unstructured data sources. These sources can include social media posts, customer reviews, and support tickets.
NLP enables businesses to gain valuable insights from the vast amount of textual information they can lay their hands on. Sentiment analysis, for example, helps companies gauge customer satisfaction and respond to feedback promptly. Businesses can understand customer sentiment, identify emerging trends, and adapt their strategies by analyzing customer interactions.
Descriptive analytics
As its name suggests, descriptive analytics describes past events and patterns. It involves taking raw historical data from minutes ago or years ago and presenting an easily understandable and accurate view of past behaviors.
Businesses gain valuable insights into how past patterns might influence the future by analyzing what has happened. Descriptive analytics is also widely used across various industries today.
For instance, businesses leverage it to understand customer buying patterns, track sales week-over-week, analyze historical cost-to-serve data, identify supply chain patterns, gain insights into financial performance, and much more. By harnessing descriptive analytics, organizations can make data-driven decisions based on a comprehensive understanding of their past performance.
Prescriptive analytics
Prescriptive analytics represents the newest evolution in the field of data analytics. It goes beyond descriptive and predictive analytics by revealing possible outcomes and prescribing courses of action.
Moreover, it provides insights into why those outcomes might occur. The main question that prescriptive analytics answers is, “What should we do?”
Even though prescriptive analytics is a relatively new form of analytics, it has already demonstrated immense potential for optimizing business processes and decision-making. Larger retail companies, for example, successfully use prescriptive analytics to:
- Enhance customer experiences
- Optimize production processes
- Improve purchasing and inventory management in the supply chain
- Ensure that the right products are delivered at the right time
Prescriptive analytics plays a vital role in the stock market by recommending optimal buying or selling points to maximize profitability. Prescriptive analytics models can also provide insights into when and where to buy or sell securities by considering multiple variables and data points. This helps investors make informed decisions and optimize their returns.
Edge computing and real-time analytics
In the era of real-time decision-making, edge computing and real-time analytics have become indispensable. Edge computing brings computation and data storage closer to the source, reducing latency and enabling faster analysis.
Real-time analytics process data as it arrives, providing businesses with up-to-the-minute insights. Industries like logistics and transportation rely on real-time analytics to optimize route planning and fleet management.
Retailers leverage real-time data to personalize customer experiences and make on-the-spot inventory decisions. Edge computing and real-time analytics empower businesses to respond swiftly to changing circumstances and capitalize on immediate opportunities.
Why is data analytics important for a business?
In various industries, data analytics is revolutionizing decision-making processes, enhancing operational efficiencies, and improving customer satisfaction. Businesses utilizing the power of data analytics gain a significant advantage in today’s competitive landscape, ensuring long-term success and growth. St. Bonaventure University offers a 100% online master of science in business analytics that teaches students to fully harness the power of data methods and technologies to strategize and solve challenges in their workplace. Here are some of the more general observations on how data analytics help businesses.
Personalized customer experience
In business, data analytics allows you to gain valuable insights into your customers’ behaviors. It also makes it easy to understand their preferences and needs. In this regard, some of the customer data you may want to analyze includes:
- Purchase history
- Browsing patterns
- Demographic information
These pieces of data allow for easier personalization of the customer experience in a highly targeted and tailored manner. Let’s take, for instance, running an e-commerce company.
In this regard, you can leverage data analytics to offer personalized product recommendations to individual customers. And the potential benefits of personalized product recommendations are enormous. You can expect higher engagement, better conversion rates, and increased customer satisfaction.
To stay ahead of the curve, try to understand your customers’ preferences. Most importantly, anticipate their needs to create a seamless and delightful experience that fosters loyalty and drives long-term customer relationships.
Informed business decision-making
Data analytics provides organizations with a wealth of insights that support informed decision-making. With these insights, you can be best placed to identify trends and patterns if you can correctly analyze both historical and real-time data.
It doesn’t end there. You will also be best placed to spot correlations that influence various aspects of your operations.
For example, if you’re in the retail business, you can use data analytics to optimize your inventory management. To do this, you will need to analyze sales data and look into demand forecasts and market trends.
This data-driven approach allows for stocking the right products in the right quantities. This can help minimize inventory costs. It can also be an effective means to meet your customers’ demands.
And what’s more, data analytics allows you to assess the impact of different strategies, campaigns, and initiatives. With the observations from analyzing performance metrics and KPIs, you can make data-driven decisions that drive growth, efficiency, and profitability.
Enhanced operational efficiency
Data analytics is instrumental in optimizing operational efficiency and streamlining business processes. Organizations can identify bottlenecks, eliminate inefficiencies, and drive continuous improvement by analyzing operational data.
For example, manufacturers leverage data analytics to implement predictive maintenance, which optimizes equipment performance by predicting failures and scheduling repairs proactively. This approach can help reduce downtime.
It also maximizes productivity and lowers maintenance costs. Moreover, data analytics enable businesses to optimize supply chain management.
They can achieve this through careful analysis of data from multiple sources, including suppliers, logistics partners, and customer demands. With insights into demand patterns, organizations can improve inventory management. They can also minimize stock outs and ensure timely delivery, thereby enhancing customer satisfaction and operational efficiency.
Mitigation of risks and handling of setbacks
Every business faces risks and setbacks, whether they are operational, financial, or external factors beyond its control. Data analytics plays a vital role in identifying, assessing, and mitigating risks, enabling organizations to handle setbacks more effectively.
Through advanced analytics techniques, businesses can analyze historical data, detect anomalies, and predict potential risks. For instance, financial institutions leverage predictive analytics to identify early signs of fraudulent activities, mitigating potential losses and safeguarding their reputation.
Data analytics also facilitates scenario analysis and simulation, allowing businesses to model different outcomes and evaluate the potential impact of various risk mitigation strategies. This proactive approach empowers organizations to make informed decisions, implement preventive measures, and respond swiftly to minimize the impact of unforeseen events.
Enhanced security
Did you also know that data analytics can also help improve your workplace security landscape? Well, it does that too.
Data security is a critical concern for every business with operations online—even SMBs. Here’s where data analytics fits in nicely. It can help you detect threats.
Since it allows you to analyze vast amounts of data in real-time, it can also help you prevent and respond to security threats promptly. To achieve this, you only need to employ advanced analytics.
With proper execution, you can monitor network traffic, detect unusual activities, and identify potential security breaches. For instance, anomaly detection algorithms can flag suspicious patterns that may indicate a cybersecurity attack, allowing you to take immediate action and safeguard your data and systems.
Data analytics also facilitates compliance with data privacy regulations by providing insights into data access and usage. By monitoring data flows and enforcing data governance policies, businesses can ensure they handle sensitive customer information responsibly and maintain trust with their stakeholders.
What are the challenges and considerations of data analytics?
The advancements in data analytics are undoubtedly transformative. However, to maximize their benefits, businesses must navigate certain challenges and considerations. Here’s a quick summary of the key areas:
Data privacy and security
As you collect and analyze vast amounts of data, do not overlook data privacy and security. In fact, they become paramount.
Check to ensure that you fully adhere to data protection regulations. Most importantly, implement robust security measures and adopt best practices to safeguard sensitive customer information. Here are some of the most effective strategies you may want to apply for data privacy and security:
- Implementing encryption techniques to secure data both in transit and at rest
- Conducting regular security audits and vulnerability assessments to identify potential risks
- Establishing access controls and user permissions to limit data accessibility
- Providing clear and transparent privacy policies to gain customer trust
Ethical implications
The increasing reliance on data analytics does raise ethical concerns regarding issues such as privacy, bias, and the responsible use of technology.
To build a solid foundation of trust with your customers and maintain societal responsibility, you must navigate these ethical considerations. Here are a few considerations you may find helpful:
- Ensuring data anonymization and aggregation to protect individuals’ privacy
- Regularly auditing algorithms to identify and mitigate biases
- Establishing ethical guidelines and frameworks for AI and machine learning usage
- Promoting transparency and accountability in data analytics practices
- Ensuring continuous learning and adaptability
Data quality and integration
Data analytics is only as reliable as the data it is based on. Just like other businesses, when dealing with multiple data sources, you may face challenges related to data quality, integration, and interoperability. Here are some recommendations to address data quality and integration challenges:
- Implementing data cleansing and validation processes to ensure data accuracy
- Establishing data governance practices and standards for consistent data quality
- Investing in data integration tools and platforms to centralize and unify data sources
- Employing data quality metrics and monitoring systems to identify and rectify issues
What are the future trends and opportunities in data analytics?
As we look ahead, the world of data analytics holds immense potential for future innovations. Here are some emerging trends and opportunities to watch for:
Emerging technologies in data analytics:
- Quantum computing: Quantum computing’s computational power has the potential to revolutionize complex data analysis. This enables businesses to solve previously unsolvable problems.
- Blockchain: Blockchain technology can enhance data security, transparency, and integrity in analytics. It achieves this by providing immutable and decentralized data storage and verification.
- Explainable AI: Explainable AI aims to provide transparency and interpretability in AI models. This can enable businesses to understand the decision-making process and build trust.
-
- Internet of things (IoT): The internet of things (IoT) generates vast amounts of data from interconnected devices and sensors. Integrating IoT data with data analytics enables businesses to gain valuable insights into real-time operations, customer behavior, and environmental factors.
- Predictive analytics: Predictive analytics, as earlier explained, uses historical data, statistical algorithms, and machine learning to forecast future trends and outcomes. It aims to help businesses make data-driven predictions and proactive decisions.
Data analytics in industry verticals:
Data analytics is reshaping various industries, and we can expect to see further advancements in the following sectors:
- Healthcare: Predictive analytics and AI-powered diagnostics can lead to personalized treatment plans and improved patient outcomes.
- Retail: Enhanced customer analytics and demand forecasting can optimize inventory management and personalize shopping experiences.
- Finance: Advanced analytics can improve risk assessment, fraud detection, and investment strategies.
- Manufacturing: AI-driven predictive maintenance and real-time analytics can optimize production processes and minimize downtime.
Final thoughts
Amidst technological advancements, data analytics has emerged as a cornerstone of modern business operations. The integration of cutting-edge technologies like AI, automation, and machine learning has empowered organizations to tap into the full potential of their data.
Looking ahead, the future of data analytics shines even brighter, propelled by emerging technologies and industry-specific applications that pave the way for innovation and growth. It is essential for businesses to wholeheartedly embrace these advancements not just to survive but to thrive in the dynamic landscape of data-driven decision-making.